Back to Blog
April 6, 20258 min read
How to Measure Lead Quality with AI: A Comprehensive Guide
Lead AnalysisShare on X

The intersection of artificial intelligence and lead generation has created powerful new opportunities for businesses to identify, evaluate, and prioritize leads with unprecedented accuracy. This comprehensive guide explores how AI is transforming lead quality measurement and provides actionable strategies for implementing these solutions in your organization.
Why Traditional Lead Scoring Falls Short
Traditional lead scoring methods typically rely on static rules and manual assessments, which present several limitations:
- Limited data processing capacity: Humans can only analyze a fraction of available data points
- Subjective evaluations: Inconsistent scoring across different team members
- Slow adaptation: Static models don't quickly adjust to market changes
- Inability to detect subtle patterns: Missing valuable correlation signals that indicate high-quality leads
The AI Advantage in Lead Quality Measurement
Artificial intelligence transforms lead quality assessment through:
1. Predictive Lead Scoring
AI algorithms analyze historical conversion data to identify patterns that correlate with successful outcomes. These systems can:
- Process hundreds of variables simultaneously
- Identify non-obvious indicators of lead quality
- Continuously improve accuracy through machine learning
- Provide probability-based conversion predictions
AI excels at tracking and analyzing prospect behaviors across multiple touchpoints:
- Website interaction patterns (page visits, time on site, scroll depth)
- Content engagement (downloads, video views, webinar attendance)
- Email response metrics (open rates, click-through, response times)
- Social media engagement with your brand
NLP capabilities allow AI to extract insights from unstructured communications:
- Sentiment analysis of prospect emails and chat interactions
- Topic extraction from support inquiries
- Intent identification from form submissions
- Competitive mentions and pain points in communications
Implementing AI Lead Quality Measurement: A Step-by-Step Approach
Step 1: Audit Your Data FoundationBefore implementing AI-based lead scoring:
- Inventory available lead data across all systems
- Identify data quality issues and gaps
- Implement data collection for missing variables
- Establish data governance practices
Clearly establish what constitutes a "quality" lead for your organization:
- Conversion events (demos, trials, purchases)
- Revenue thresholds
- Customer lifetime value
- Implementation success
- Expansion potential
Choose AI solutions based on your specific needs:
- Built-in CRM AI capabilities: For organizations with limited technical resources
- Dedicated lead scoring platforms: For specialized scoring needs
- Custom AI models: For enterprises with unique requirements and data science resources
Effective AI deployment requires:
- Historical data for initial model training
- Integration with existing marketing and sales platforms
- A phased rollout approach
- Regular model validation and refinement
Transform AI scores into actionable workflows:
- Lead routing based on quality thresholds
- Personalized nurture paths for different quality segments
- Sales prioritization frameworks
- Automated follow-up sequences
Case Study: OasisAuto
OasisAuto implemented an AI-driven lead scoring system with impressive results:
- 47% increase in sales qualified lead identification
- 28% reduction in sales cycle length
- 35% improvement in conversion rates
- 22% increase in average deal size
Their success stemmed from combining predictive scoring with behavioral analysis and integrating these insights directly into their sales workflows.
Measuring the Impact of AI Lead Quality Assessment
Track these metrics to evaluate your AI lead scoring effectiveness:
- Lead-to-opportunity conversion rates
- Sales cycle velocity
- Resource allocation efficiency
- Revenue per lead
- Sales team feedback and adoption
Ethical Considerations
When implementing AI for lead quality measurement:
- Ensure transparency in how leads are scored
- Avoid bias in training data and algorithms
- Maintain compliance with privacy regulations
- Preserve the human element in final decision-making
Conclusion
AI is revolutionizing how businesses measure and respond to lead quality. Organizations that effectively implement these technologies gain significant competitive advantages through improved efficiency, higher conversion rates, and more strategic resource allocation. By following the framework outlined in this guide, you can transform your lead quality measurement and create a more predictable, profitable revenue engine.
Share this article
Related Articles
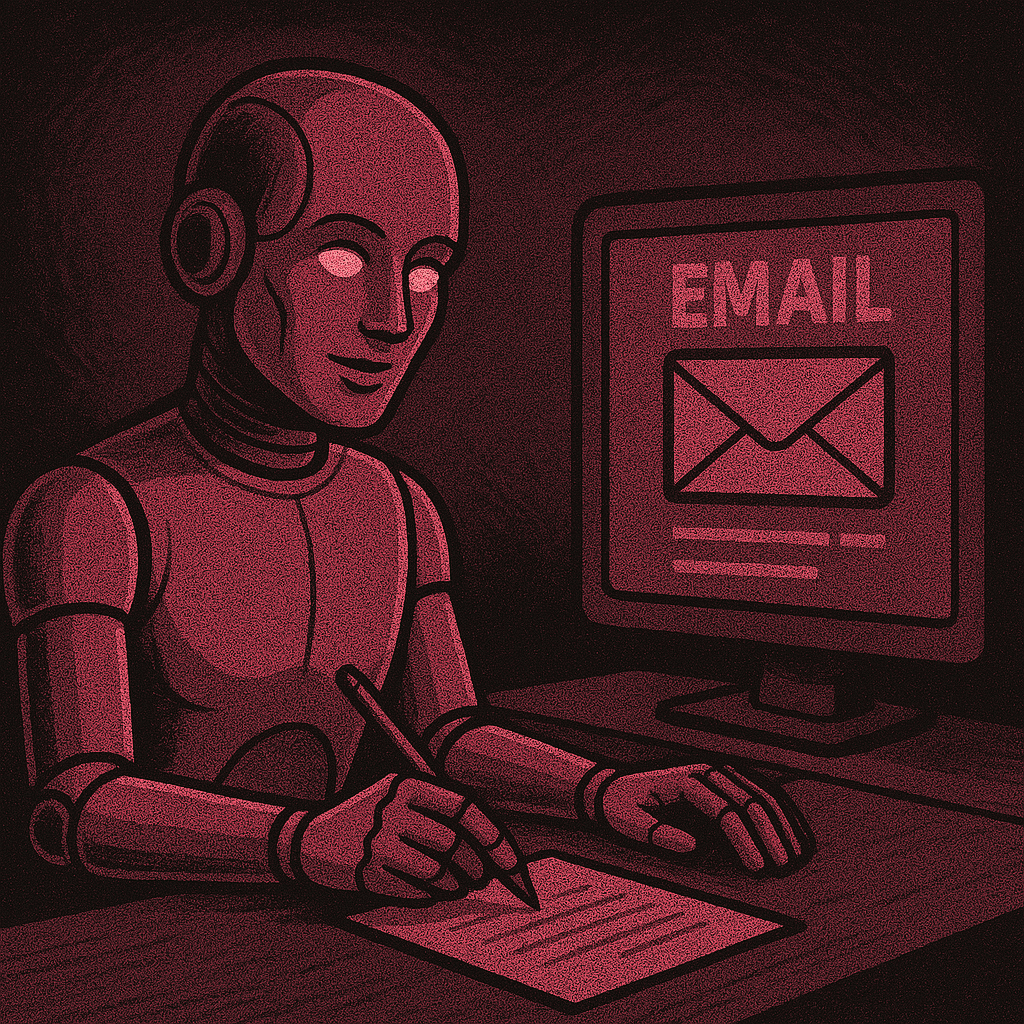
Cold Calling9 min read
How a Cold Calling AI Agent Turns Cold Leads into Hot Prospects
Discover how AI-powered cold calling agents can transform your outreach strategy, qualify leads automatically, and fill your sales pipeline without the tedious grunt work of manual dialing.
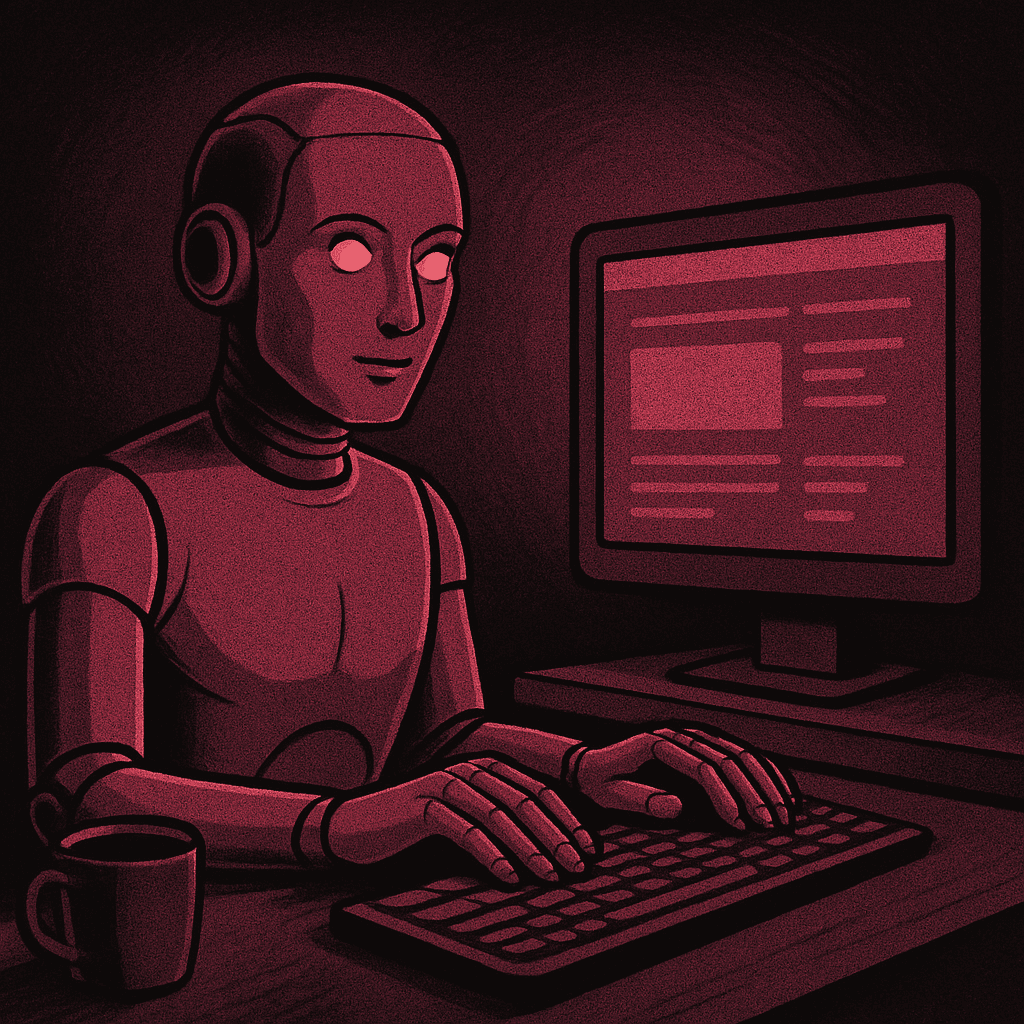
Sales Automation7 min read
The Future of Sales: How AI is Automating the Sales Process
Explore how artificial intelligence is transforming sales automation, from lead scoring to personalized outreach, and learn how to implement these technologies in your sales strategy.